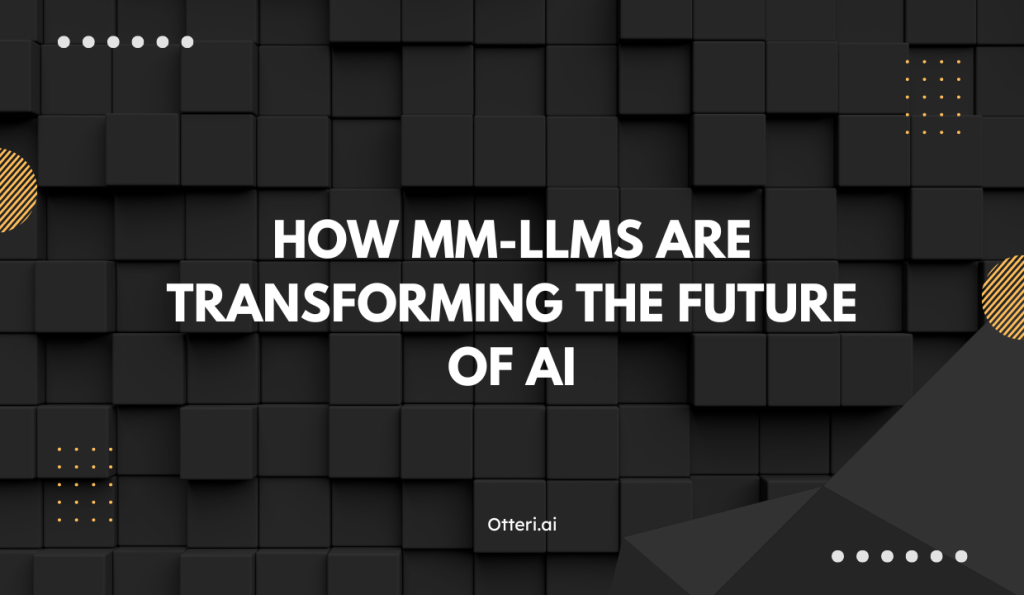
How MM-LLMs are reshaping artificial intelligence by processing text, images, and audio together. Learn about their real-world impact and future potential. In recent years, large language models (LLMs) have made significant progress in understanding and generating text that resembles human language. However, human communication extends beyond just language. We use images, sounds, gestures, and even video to express ideas. This is where multimodal large language models (MM-LLMs) step in, pushing the boundaries of what artificial intelligence can understand and produce.
MM-LLMs can process and reason across various types of inputs — including text, image, audio, and sometimes even video — all at once. This cross-modal understanding is redefining how AI can assist in education, healthcare, creative industries, and beyond.
What Makes MM-LLMs Different?
Traditional LLMs, such as GPT-3 or early versions of BERT, are limited to textual inputs. They can read and respond to written prompts but cannot interpret a photo, analyze a voice note, or describe a scene from a video.
MM-LLMs, on the other hand, are trained on multimodal datasets. They combine different neural architectures and encoders to understand multiple forms of data simultaneously. This makes them more context-aware, flexible, and suitable for tasks that require a richer understanding of the world.
Key Technological Advances
The recent surge in MM-LLM capabilities is due to several breakthroughs:
Multimodal Training Datasets
Tech companies are investing in large-scale datasets that include images, audio, and paired captions or transcripts. These help models learn relationships across formats.
Cross-Modal Attention Mechanisms
Models like GPT-4V and Google Gemini utilize attention mechanisms that allow information to flow between different data types. This enables better interpretation of images with accompanying text.
Unified Architectures
Instead of using separate models for each data type, unified architectures now process all modalities within a shared framework. This leads to improved performance and more natural responses.
Scaling Capabilities
As computational power increases, so does the capacity to train deeper and more complex models that handle more data types with higher accuracy.
Practical Applications of MM-LLMs
MM-LLMs are not just theoretical advancements. They are being deployed across industries in ways that were not possible just a few years ago.
Education
Students can ask questions using voice, text, or even drawings. The model can respond with explanations, visuals, and interactive examples.
E-commerce
Users can upload images of products to find similar styles, get detailed descriptions, and even receive personalized shopping suggestions.
Healthcare
MM-LLMs are being explored for diagnostic assistance by interpreting a combination of medical images, doctor’s notes, and patient symptoms.
Content Creation
Creators are using these models to generate video summaries, design ideas, or audio narration based on written scripts or visual inputs.
Customer Support
AI agents can now understand customer screenshots, photos of products, and voice queries — making support faster and more accurate.
Leading MM-LLM Models and Tools
Several models and platforms are setting new benchmarks in the field:
GPT-4V (OpenAI): Capable of image understanding alongside text-based interactions.
Gemini (Google DeepMind): Trained to handle text, image, audio, and video data in an integrated manner.
ImageBind (Meta): Focused on connecting diverse sensory data types like depth, thermal imaging, and motion.
Flamingo (DeepMind): Designed for few-shot learning with images and text.
CLIP and DALL·E: While not full MM-LLMs, they enable strong connections between text and images for retrieval and generation.
These tools are being used in products ranging from AI chat assistants to enterprise-level automation systems.
Challenges to Consider
Despite their promise, MM-LLMs are not without limitations.
Data Availability and Bias
Multimodal datasets are harder to curate and often contain bias. Poor quality or unbalanced data can lead to inaccurate or unfair outputs.
Computational Costs
These models are large and require significant computing resources to train and run effectively, making them less accessible for smaller organizations.
Interpretability
As with many deep learning systems, MM-LLMs are often black boxes. Understanding how they reach specific conclusions remains a challenge.
Ethical and Privacy Concerns
Handling multimodal data, especially user-uploaded images or audio, raises concerns around consent, storage, and misuse of personal information.
What’s Ahead for MM-LLMs?
The future of MM-LLMs is highly promising. Several trends suggest rapid development:
Improved fine-tuning methods will allow these models to become more domain-specific.
Smaller, more efficient MM-LLMs will make it feasible to run multimodal models on edge devices.
Real-time interaction with voice, gesture, image, and video input will become more seamless.
Open-source contributions will democratize access to advanced MM-LLM technologies.
As more developers and companies adopt these models, innovation will likely accelerate.
Conclusion: Why MM-LLMs Matter
Multimodal large language models are not just an upgrade to existing AI systems — they represent a fundamental shift. By combining the ability to read, see, and listen, MM-LLMs are offering a more human-like understanding of context.
At Otteri.ai, we believe in staying at the forefront of this shift. Whether you’re building smarter chat interfaces, educational tools, or automation solutions, MM-LLMs open up possibilities that were once thought impossible. As technology matures, its real-world impact will continue to grow.
Call to Action
Ready to explore the power of multimodal AI?
Visit otteri.ai to learn how you can integrate MM-LLM capabilities into your next project.