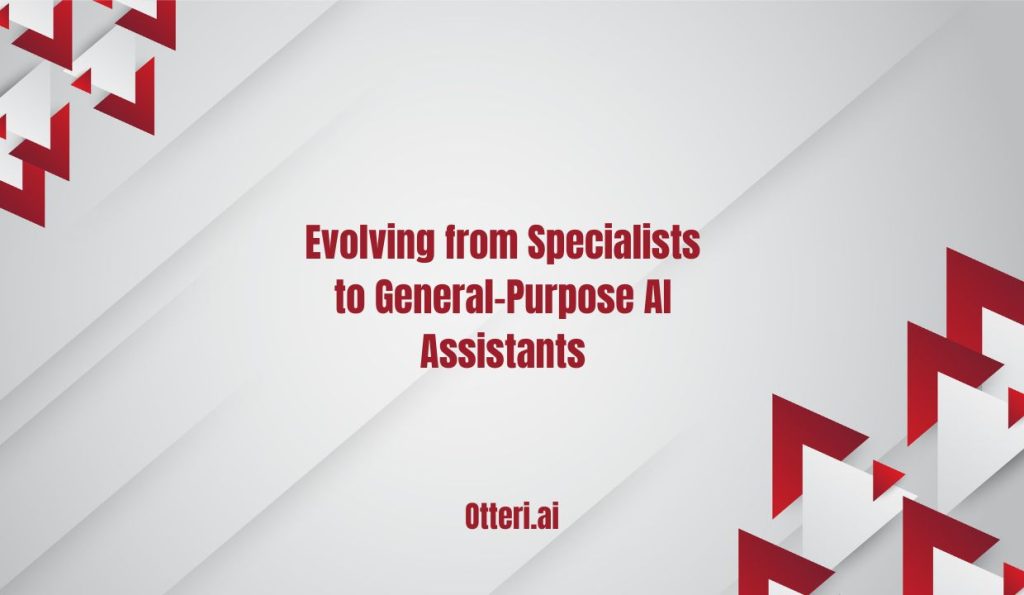
Explore how multimodal foundation models are evolving from task-specific tools into powerful general-purpose AI assistants. Discover their growing capabilities, real-world applications, and their transformative impact across industries.
Artificial Intelligence is experiencing a significant evolution, with multimodal foundation models at the forefront. These large-scale AI systems can understand and generate information across multiple formats—text, images, audio, and video. Initially developed for specialized tasks, these models are now being trained and scaled to function as general-purpose AI assistants capable of handling a wide range of functions. Their development is not only revolutionizing industries but also reshaping workflows and redefining how humans interact with machines.
Understanding Multimodal Foundation Models
Multimodal foundation models are advanced neural networks trained on vast, diverse datasets. Unlike earlier models restricted to a single modality—such as text-only or image-only models—these systems integrate and interpret data across formats. For instance, a multimodal model can answer a text-based question by referring to an image and then responding with both textual explanation and visual modification.
These models leverage transformer architectures and self-supervised learning techniques to build complex cross-modal representations. As a result, they can generalize across tasks and domains far more effectively than traditional, specialized models.
From Task-Specific Tools to Versatile AI Assistants
Early foundation models were narrow in scope. A model might have been highly accurate in captioning images or identifying visual elements but lacked the versatility to perform tasks like sentiment analysis or creative content generation. Over time, researchers introduced multitask learning strategies and trained models on diverse datasets. This expansion allowed these models to develop broader capabilities.
Today’s multimodal models—such as OpenAI’s GPT-4 with Vision, Google’s Gemini, and Meta’s LLaVA—are capable of summarizing documents, analyzing images, interpreting charts, recognizing speech, and more. Trained across various data types, these systems now act as comprehensive assistants, able to handle complex, multimodal queries with ease.
Key Features of General-Purpose Multimodal Assistants
The transition from narrow tools to versatile assistants has unlocked several transformative features:
Cross-Modal Reasoning
These models can interpret and synthesize information across formats. For example, a user can upload a chart and ask for a written summary or submit a photo for design feedback.Context Awareness
By analyzing both visual and textual inputs, multimodal models provide more accurate and nuanced responses.Zero-Shot and Few-Shot Learning
They can perform new tasks with little to no prior examples, minimizing the need for fine-tuning.Natural Interaction
Users can interact using text, voice, or images—enabling seamless, intuitive communication.Scalability and Adaptability
These models are flexible enough to be applied across various sectors, including healthcare, education, marketing, and more.
Real-World Applications
The practical applications of multimodal models are expansive and growing:
Healthcare
Doctors can upload X-rays and receive summaries of potential diagnoses. Voice-based symptoms can be analyzed alongside patient histories for informed recommendations.Education
Educators can convert textbook content into visual presentations, auto-generate quizzes, and simplify complex diagrams for better student comprehension.Marketing and Content Creation
Brands can turn written prompts into visual social media content, analyze consumer feedback, and generate advertising scripts from market data.Software Development
Developers can use AI to convert design mockups into code or troubleshoot bugs based on screenshots.Customer Support
AI can interpret screenshots or audio from customers and provide automated resolutions or escalate cases when necessary.
The Architecture Behind the Intelligence
At the core of these capabilities lies the transformer architecture, equipped with multimodal encoders and decoders. These components allow the model to align and understand different data types within a shared embedding space. This unified representation enables seamless translation and interpretation across modalities.
For instance, if a user uploads an image of a damaged machine and asks, “What’s wrong with this?”, the model can visually inspect the photo, compare it against known issues, and provide diagnostic suggestions—all within a single interaction.
Challenges and Ethical Considerations
Despite their promise, general-purpose multimodal models face notable challenges:
Bias and Fairness
If training datasets include biased content, the model may replicate or amplify these biases.Misinformation
The ability to generate highly realistic content raises the risk of deepfakes and misleading outputs.Data Privacy
Training such models requires large amounts of data, sparking concerns over how that data is collected and used.Interpretability
Understanding how these models arrive at certain decisions remains difficult, especially in critical fields like healthcare and law.
Ensuring responsible development involves transparency, regulation, and a focus on ethical AI practices that prioritize human values.
The Future: Human-AI Collaboration
The future of multimodal models lies not in replacing human expertise, but in enhancing it. These AI systems are poised to become creative collaborators, analytical partners, and problem-solving assistants across industries.
Imagine a designer brainstorming visual concepts through AI-generated sketches, or a lawyer drafting legal documents with assistance from AI that summarizes case law from PDFs and images. In filmmaking, directors could use rough scene sketches to auto-generate scripts and camera directions.
By merging human creativity with machine efficiency, multimodal models serve as a bridge between natural human expression and computational understanding.
The evolution of multimodal foundation models from narrow tools to general-purpose assistants is a landmark in the development of AI. By integrating various types of input and providing meaningful, multimodal output, these models are redefining what human-machine interaction can look like. Their capacity to understand, reason, and respond across text, image, audio, and video makes them invaluable across countless domains.
As we move forward, the focus must remain on responsible innovation, ensuring these powerful tools are used ethically and transparently. With the right guardrails, multimodal AI can become one of the most impactful technologies of our time.